Future of AI and Machine Learning: Trends and Impacts
Technology is changing everything around us, and two of the most talked-about topics lately are Artificial Intelligence (AI) and Machine Learning (ML).
It might sound like something out of a sci-fi movie, but these technologies are part of our daily lives: recommending movies on Netflix, adjusting Instagram feeds, and even assisting in medicine. The best part? You don’t need to be a scientist to understand the basics!
This article is a complete, no-nonsense guide to help you understand what AI and ML are, where they are used, the challenges they present, and even how to start learning more about them.
What is Artificial Intelligence?
Advertising
Remember those movies where robots seem to think on their own? That’s what we call Artificial Intelligence, but in real life, things are a bit different.
AI is essentially a set of technologies that enable machines to “think” or make decisions similar to humans. They learn from data, analyze information, and can perform tasks automatically.
A bit of history
Advertising
The term “Artificial Intelligence” emerged in the 1950s, but back then, it was just a theoretical concept. The AI boom happened in recent years with the advancement of computers and the increase in available data.
Today, AI is everywhere: from voice assistants like Alexa to self-driving cars.
Practical examples of AI
- Virtual assistants: Siri, Google Assistant, and Alexa.
- Recommendations: YouTube, Netflix, and Spotify suggesting what to watch or listen to.
- Security: Cameras that detect movement or recognize faces.
What is Machine Learning?
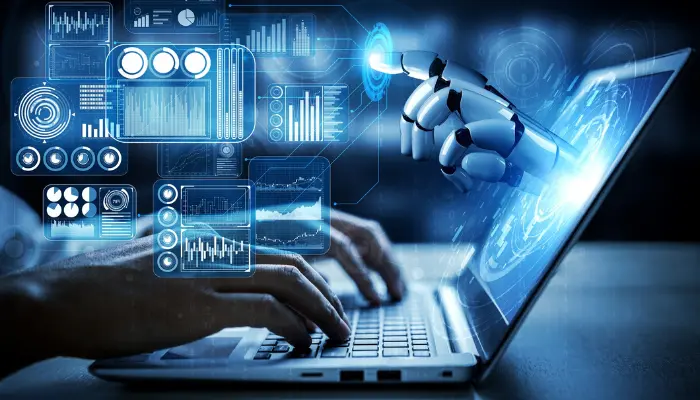
Now that you understand what AI is, let’s talk about Machine Learning (ML). ML is a part of AI, like its “right-hand tool.”
The difference is that, in ML, machines learn independently from the data they receive without needing someone to program every detail.
How does it work?
Imagine you want to teach a machine to identify whether a photo shows a dog or a cat. In ML, you provide several photos, labeling which ones are cats and which are dogs.
Over time, the machine “learns” to identify them on its own. This happens through algorithms (a step-by-step process that machines follow).
Types of learning in ML
- Supervised: The machine learns from examples you provide.
- Unsupervised: The machine tries to understand patterns in the data on its own.
- Reinforcement: The machine learns through trial and error, like in a game.
Difference Between Artificial Intelligence, Machine Learning, and Deep Learning
If you think AI, Machine Learning, and Deep Learning are the same, don’t worry—it’s a common misconception! They’re connected but have unique distinctions. Let’s break it down:
- Artificial Intelligence (AI): The broadest concept that encompasses all technologies involving machines that “think” or make decisions like humans. Anything that simulates intelligence, from a robot to a virtual assistant, falls under AI.
- Machine Learning (ML): A subset of AI where machines learn independently by analyzing data. Instead of programming every step, you train the machine with examples, and it identifies patterns.
- Deep Learning (DL): The “advanced level” of ML. Inspired by the human brain, it uses neural networks to solve complex problems like facial recognition and automatic translation.
A simple analogy: AI is like a school, ML is a specific class, and Deep Learning is the genius professor who solves the toughest problems with ease.
Main Algorithms in Machine Learning
Now let’s dive into the “engines” of Machine Learning: the algorithms. These are the methods machines use to learn and make decisions.
Although it might sound technical, I’ll explain in a simple way. ML algorithms are divided into three main categories: supervised, unsupervised, and reinforcement learning. Let’s explore:
Supervised Algorithms
In supervised learning, you provide examples for the machine to learn. It’s like teaching a child to identify fruits by showing them images and saying, “this is an apple,” or “this is a banana.”
The machine uses these examples to predict or classify information in the future.
- Linear Regression: Perfect for predicting numerical values, like the price of a house based on size or location. It finds the straight line that best fits the data.
- Decision Trees: Like a diagram of “yes” or “no” questions. Commonly used for classification tasks, such as predicting whether a customer will buy a product or not.
Unsupervised Algorithms
In unsupervised learning, the machine works without labels or predefined answers. It examines the data and tries to identify patterns on its own, like solving a puzzle without the final picture.
- K-means (Clustering): Divides data into groups. For example, it can separate customers by purchasing behavior without you having to define the groups beforehand.
- PCA (Principal Component Analysis): Great for summarizing information. It reduces the complexity of data, keeping only the most relevant parts, like a summary of a large book.
Reinforcement Learning
This is the most “game-like” of the algorithms. It works through trial and error, learning from rewards and penalties, like training a dog to fetch a ball.
- Example: In robotics, the algorithm learns how to make a robotic arm pick up objects without breaking them. It’s also used in games, where the machine learns strategies by playing and improving over time.
These algorithms are the driving force behind Machine Learning. Each has its role and shines depending on the problem you want to solve. Whether predicting, classifying, or uncovering patterns, they’re always ready to impress!
Applications of Artificial Intelligence and Machine Learning
Now that you understand the basics, it’s time to see where Artificial Intelligence and Machine Learning are being used. Spoiler: practically everywhere!
From healthcare to retail, these technologies are game-changers, making everything more efficient, faster, and often safer. Let’s explore their main areas of application.
In Healthcare
AI and ML are revolutionizing healthcare, helping doctors and patients in ways that once seemed impossible.
- Quick and accurate diagnoses: AI algorithms can identify diseases, like cancer or heart problems, by analyzing tests in seconds, often with greater precision than humans.
- Robot-assisted surgeries: Robots equipped with AI assist in delicate surgeries, providing greater precision and reducing risks.
- Epidemic prediction: ML systems analyze large datasets, such as travel patterns and climate changes, to predict disease outbreaks before they occur.
In Retail
If you’ve ever felt like an online store knew what you wanted before you did, you can thank AI.
- Personalized recommendations: Machine Learning analyzes your browsing and purchase history to suggest products you’re likely to love.
- Smart inventory management: AI algorithms predict product demand, avoiding overstock or shortages.
- Customer experience: Virtual assistants and chatbots make customer service faster and more efficient.
In Industry
Automation is taking over the industrial sector, and AI and ML are behind much of it.
- Predictive maintenance: Machines equipped with AI detect signs of failure before problems occur, preventing unexpected downtimes.
- Process automation: AI-powered industrial robots perform repetitive and hazardous tasks, reducing costs and boosting productivity.
- Quality control: Algorithms identify defects in products with greater precision than the human eye.
In Education
Education is also being transformed, with AI tools personalizing the learning experience for each student.
- Adaptive platforms: AI systems adjust content and difficulty based on each student’s performance, ensuring no one is left behind.
- Teacher support: Tools analyze class progress, identify students needing extra help, and even create personalized exercises.
- Accessibility: AI solutions, like automatic captions and translators, make learning more inclusive.
In Security
Whether protecting people or data, AI and ML are making the world safer.
- Intelligent surveillance cameras: AI systems can identify suspicious movements, faces, or vehicle plates in real time.
- Crime prevention: Algorithms analyze historical data to predict high-risk areas and times, aiding in preventative measures.
- Cybersecurity: AI detects patterns of online attacks and responds automatically to protect systems and data.
These are just some of the areas where AI and ML are being used to achieve amazing things. And the best part? This is just the beginning.
Every day, new applications are emerging, showing how these technologies can improve our lives in nearly every aspect!
Ethics and Challenges in AI and ML
Artificial Intelligence and Machine Learning may seem like a dream come true, right? But not everything is perfect. These technologies also bring challenges, both on the technical and ethical fronts.
After all, the more powerful the tools, the greater the responsibility to use them correctly.
Ethical Concerns
The biggest ethical challenge in AI and ML is ensuring they are used for good, without harming people or groups. Here are the key points to watch out for:
Algorithmic Bias:
Algorithms are only as fair as the data they receive. If these data contain biases (such as discrimination based on race, gender, or class), the decisions made by AI will also be biased.
Example: Recruitment systems that favor men because historical data show most hires were male.
Privacy:
AI and ML require vast amounts of data to perform well, raising the question: how secure is our information? With so much data being collected, there’s a significant risk of leaks or unauthorized use.
Impact on Employment:
Automation and robots are replacing certain professions, particularly those involving repetitive tasks. Without proper planning for worker retraining, this could lead to mass unemployment.
Technical Challenges
Beyond ethical issues, technical challenges also hinder the effective development and use of these technologies.
- Data Quality: For AI to function well, models need to be trained on large volumes of reliable and well-organized data. Poorly collected or incomplete data can lead to incorrect decisions.
- Interpretability: Sometimes, even experts can’t explain how AI arrived at a particular decision. This is a critical issue, especially in sensitive areas like healthcare or justice, where understanding the “why” behind a choice is essential.
Despite these challenges, it’s important to remember they are not insurmountable barriers.
With proper regulation, greater transparency, and an ethical approach, AI and ML have the potential to positively transform the world. However, achieving this requires care and responsibility from everyone involved.
Popular Tools and Languages in Machine Learning
If you’re thinking about diving into the world of AI and Machine Learning, you need to know the tools and languages that form the foundation of this field.
With these, you can create models, analyze data, and even develop complete applications—even if you’re not an expert. Let’s explore the most widely used options, whether you’re just starting or already in the field.
Main Libraries
Libraries are pre-built code collections that make your work easier. They save time and help you create ML models more efficiently. Here are the most popular ones:
- TensorFlow and PyTorch: These are the giants of Machine Learning. Primarily used by professionals, these libraries help build advanced models, such as neural networks for Deep Learning.
- Scikit-learn: Perfect for beginners, it offers simple tools to create basic models like regressions and classifications without the complexity.
Programming Languages
Choosing the right programming language makes all the difference when working with AI and ML. Two dominate the market:
- Python: The favorite for ML due to its simplicity and vast collection of libraries and tutorials. It’s ideal for both beginners and professionals.
- R: Focused more on statistics and data analysis, it’s widely used in academic research and fields that require heavy mathematical calculations.
Platforms
If you want to use AI and ML without building everything from scratch, platforms are your best choice. They offer ready-made tools to train, test, and deploy models:
- Google Cloud AI: Provides robust solutions for creating and managing ML models with seamless integration into Google’s ecosystem.
- AWS Machine Learning: Amazon’s platform is great for businesses, offering scalable tools for projects of any size.
- Microsoft Azure: Perfect for those already using Microsoft’s ecosystem, with numerous integrated AI options.
These tools and languages are the foundation of any Machine Learning project. The right choice depends on your goals and skill level, but the most important step is to start and practice.
The more you explore, the easier it will be to create your own solutions!
The Future of Artificial Intelligence and Machine Learning
The future of Artificial Intelligence and Machine Learning is as exciting as it is challenging. These technologies are evolving rapidly and promise to transform our world even further.
But with great advances come great responsibilities. Let’s explore the key trends and impacts shaping tomorrow.
Trends to Watch
What’s on the horizon for AI and ML is breathtaking. New ideas and technologies are being developed to make these fields even more powerful and accessible:
- Generative AI: Tools like ChatGPT create text, images, music, and videos automatically, revolutionizing entertainment, marketing, and art.
- Auto-ML: Platforms that let you build AI models without being an expert, making the technology accessible to everyone.
- Quantum Computing: Promises to accelerate complex calculations, bringing revolutionary advancements to AI.
Impacts Ahead
With so much innovation, the impacts will be massive, both in the market and in society. Here are some of the key changes we can expect:
- New professions and markets: AI and ML will create jobs in fields that don’t yet exist, such as AI ethics specialists and autonomous learning system architects. Companies adopting these technologies will gain a significant competitive edge.
- Solutions to global problems: Imagine using AI to address challenges like climate change, optimize natural resources, or develop personalized treatments for diseases. These advancements could turn seemingly impossible challenges into practical solutions.
The future of AI and ML is full of possibilities but also demands caution. Society must be prepared to address ethical issues, establish regulations, and adapt to the impact on the workforce.
When used responsibly, the potential of these technologies is limitless and could transform the world in ways we can’t yet imagine.
How to Learn Artificial Intelligence and Machine Learning?
Interested in diving into the world of AI and Machine Learning but don’t know where to start? Don’t worry—you don’t need to be a programming genius! With dedication and the right resources, anyone can learn.
Here are some practical tips to help you take your first steps.
Choose an Online Course
Today, there are countless courses available, from free options to paid ones, ranging from basic to advanced. Here are a few recommendations:
- Free: Platforms like Coursera, edX, and YouTube offer introductory courses that are perfect for beginners.
- Paid: If you’re looking for something more comprehensive, courses on Udemy, DataCamp, or Alura are great investments.
Practice with Projects
Learning AI and ML isn’t just about theory—you need to get hands-on experience.
- Start with simple projects, like creating a model to predict house prices based on size or location.
- Use platforms like Kaggle or Google Colab, which provide ready-to-use datasets and environments for practice.
Join Communities
Connecting with others who are learning or already working in the field can greatly accelerate your progress.
- Online forums: Stack Overflow is perfect for resolving technical questions.
- LinkedIn and Discord groups: Find people sharing tips, tutorials, and even collaborative project opportunities.
Invest in Certifications
Certifications are a great way to validate your knowledge and stand out in the job market.
- Google AI, AWS, and Microsoft Azure: These platforms offer globally recognized certifications that demonstrate your practical skills in AI and ML.
Explore Additional Resources
Beyond courses and projects, read articles, watch YouTube lectures, or follow professionals in the field on LinkedIn. Continuous learning and staying updated are essential in this rapidly evolving area.
With these steps, you’re ready to begin your journey into Artificial Intelligence and Machine Learning. The key is practice and curiosity—always explore and learn more. So, why not take the first step today?
AI and ML are already part of our lives and will only grow. Whether you want to use these technologies at work, understand more about them, or simply keep up with global changes, the important thing is to start.
Are you ready to explore this universe? Share your thoughts, and let’s continue this conversation!